Point Estimates
To start with our chapter on confidence intervals we initiate with the topic of point estimation. Point estimation is an area of statistics which tries to provide a best guess for a certain characteristic or parameter of a population while performing simplistic calculations. This best guess is what we call a point estimate.
Let us take a little introduction on this concept in the next section for this lesson.
What is a point estimate?
Simply said, a point estimate is a statistic. But what does that mean? A statistic is a measurable characteristic, a way to see how much of a particular attribute is contained within a sample from a population. Therefore, a point estimate is then the best guess or the best estimation of how much (or many, depending on the case) of a characteristic quantity or quality a sample has.
There are different types of estimators in statistics, but the simplest are the point estimates, and that is why we are starting with this topic today.
The advantage of a point estimate against other estimations is that it is very simple to calculate and to understand. Still, it does have some pretty important disadvantages, the main being that a point estimate does not allow the person computing the estimation to be sure if the estimation is actually representative of the population or not, since there are not that many statistical parameters taken into account while calculating a point estimate.
But you may be wondering, what does a point estimate really do? There are different kinds of point estimates, and the results they provide depend on which one you are working with. Some of the most common point estimates are the sample mean, the sample proportion and the sample standard error. On this lesson we will focus on the sample proportion point estimate.
When studying a large population with their proper behaviour and overall characteristics (depending of what population you are studying), we may be wondering about the proportion of the population which possess a certain quality or characteristic.
Therefore, we will try to estimate how much of the population has such a quality. This is what the sample proportion point estimate is all about!
In general, a point estimate is the simplest estimator that can be calculated in statistics; for the case of the populations quality as described in the paragraph above, only the sample proportion point estimate could respond the question on what proportion of the population possess a certain quality: By simply taking a sample of the population and then counting the amount of individuals in the sample which possess the desired quality, we can obtain the ratio of those who have the quality versus the whole population and thus know the proportion of success for such quality from the sample. With that proportion in mind, we can then apply it to the whole population by implying that the whole population has a certain proportion of individuals with the desired quality based on the estimation gotten from the sample.
Let us take a look at an easy point estimate example so we can explain the concept clearly:
Imagine you go to a pug rescue and shelter as a voluntary and while working with the beautiful pugs, you are in charge of separating a few of them to prepare them for a bath.
Out of the 184 pugs in the shelter, this is the group of pugs you have in one of the patios waiting for a bath, take it as a sample from your pug population.
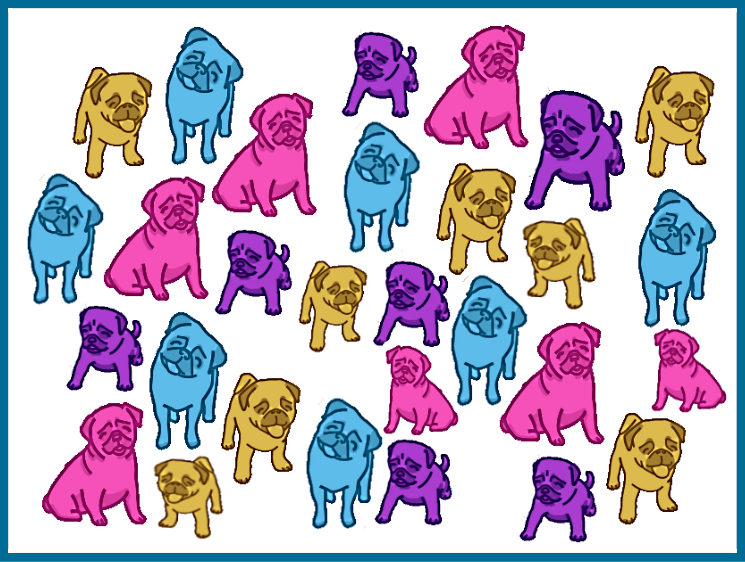
Based on this sample, what proportion of pugs in the shelter do you think are yellow?
Easy! You just count the total of pugs in your sample (in this case 29), and then count the pugs that are yellow (there are 8 yellow pugs). Therefore, you can quickly arrive to the conclusion that there are a total of 8 yellow pugs out of 29 pugs! That right there is a good estimate of the proportion of pugs that are yellow in the whole shelters population! And so, this sample proportion point estimate is 8/29 (eight out of twenty-nine).
As you can see from the example, this estimation is very easy to calculate and understand, is logical and can serve many purposes; still, as mentioned before, its biggest disadvantage is that by just obtaining such an approximate proportion of what is to be expected, we cannot assure this is a representative proportion for the whole population. We are not taking into account the sampling methods used to obtain the sample, we are not appreciating the type of the bias the sample may have (for example, what if the main caretaker selected only the young pugs, or the old ones, or the well behaved ones) and so, we cannot really know how representative the calculated estimation truly is for the whole pug shelter population.
Of course, such uncertainties can be reduced by taking care about the way a sample is taken and the size of it, or by repeating the statistical experiment (taking other samples of groups of pugs out and see if the proportion remains) as many times as we want and look at the trend of the results and their average: do they vary much from one group to the next? Have you discovered any unnoticed bias, etc.
With all this in mind, there is not doubt point estimate statistics are important since they serve as the basis for well funded observation approximations (in this case of proportions), but they are just the beginning!
So, before we go into less uncertain estimations such as interval estimates and the confidence in such intervals, let us learn about the point estimates and practice a fews problems.
How to find a point estimate?
Remember that the beauty of a point estimate, is that it consists of a single value which describes the population of interest as best as possible; therefore, it provides a quick overall view of the characteristics of a certain statistical parameter when surveying or gathering information from a population in a rapid manner.
For this lesson, we are focusing on sample proportion, and so, the point estimate definition for sample proportion goes as:
Where:
the point estimate or the sample estimate
the population portion (this is the data we are ultimately concerned onfinding)
sample size
number of successess
Remember, equation 1 is only one point estimate formula, dedicated exclusively to proportion. In short, we can define the sample proportion point estimation as the successes in a sample (those individuals with the desired trait), divided by the total number of individuals in the sample (sample size).
We will use the point estimation definition in equation 1 in the next section to answer the example problems provided.
Point estimate examples
Now let us take a look at a few example problems dedicated on finding the point estimate for you to practice. Remember that we are only calculating sample proportions (the point estimates we are focusing on today).
Example 1
If a sample experiment with 100 trials has 63 successes what is the resulting point estimate?
For this case, we have that the total number in the sample is 100 trials (), while the number of successes is equal to 63 (); therefore, using the point estimate formula we calculate:
Therefore, the point estimate of the experiment is 0.63.
Example 2
The New York Times wants to compute roughly how many Pokémon Go players there are in the US. So they sample 2500 people and ask them whether they play Pokémon Go, or not. A total of 275 people said they played Pokémon Go.
1. What is the best point estimate for the of Pokémon Go players for the sample?
For this case, we have a sample size of 2500 people, therefore ; out of the twenty-five hundred people sampled, only 275 people responded successfully to playing Pokémon Go, therefore, that is the amount of successes from the sample: .
2. If the US has a population of 320,000,000 using your data from the previous question what would be a good estimate for the number of Americans that play Pokémon Go?
In order to obtain a good estimate of the people who play Pokemon Go using the point estimate calculated in part one, we just have to multiply the total population given for the US, times the point estimate found.
Why? Let us go back to equation 1 for this: if the point estimate is equal to the number of successes in a sample, we could do reverse engineering and say, if our sample was the size of the population (in this case the given population of the US = 320,000,000), then this question is asking us to find the number of successes once we have the point estimate. Therefore:
And so, about 35,200,000 Americans play Pokemon Go based on the sample taken.
Example 3
A city survey says that after gathering the response from a sample of 5000 people in the street, they estimate that 78% of the population think that the prices of rental units are too expensive and something has to be done.
1. Knowing this, calculate how many people in the survey responded by saying rent prices are expensive:
For this case we have that the size of the sample is equal to 5000, while the sample proportion point estimate is equal to 0.78 (which comes from the 78%). Therefore, we calculate:
Therefore, a total of 3900 people in the survey responded that rent in the city is expensive.
Example 4
While trying to promote a product, a small business in a shopping mall got a sample of 300 people to try their product. Out of the 300 customers, 182 responded that the product was a success and they were interested in obtaining it once it hits the shelves.
What is the sample proportion for which the product was a success?
Therefore, the small business owners have gotten a point estimate that predicts the new product will be a success for 60.666% of the population in the shopping mall.
This is the end of our lesson, before we go, we recommend you to take a look at this lecture on point estimation. Also, this lesson on point estimation and confidence intervals is useful for you not only for the topic of today, but also for our next one, a lesson where we will be introducing confidence levels. Notice that both links talk about other types of point estimates (not only proportions) and so, they can be of aid in your independent studies to complement what you have learned here today.
We hope you have enjoyed the lesson, and we will see you in the next!