In this lesson, we will learn:
- The difference between theoretical and experimental probability
- How to calculate the number of expected outcomes using theoretical probability and number of experimental trials
- How to write the experimental probability as a fraction based off the observed results in an experiment
Notes:
- Probability for simple events means we are just looking at one probability scenario at a time (i.e. one coin flip; a single six-sided die toss; one spinner)
- There are two types of probability:
- Theoretical probability is an educated guess on what you think should or will happen ("expected" probability; based on theory)
- Experimental probability is based on an experiment and what you saw happen already ("observed" probability; happened in reality)
- The probability we have seen so far in previous lessons is based on theoretical probability. We can use theoretical probability to find the number of expected outcomes.
- Before you do an experiment, you can predict how many times an outcome will happen (how many times it should theoretically happen)
(event) = - This is based on the number of trials you will do in the experiment. A trial is each run through of the experiment--1 trial will give 1 outcome (each coin flip, each dice toss, each spinner spin)
# expected outcomes = (event) × # trials - The experimental probability is based off the observations made during the experiment and calculated once all trials are completed.
(experimental event) =
Ex. For an experiment, a coin is flipped 8 times. The results are shown in an observation table:
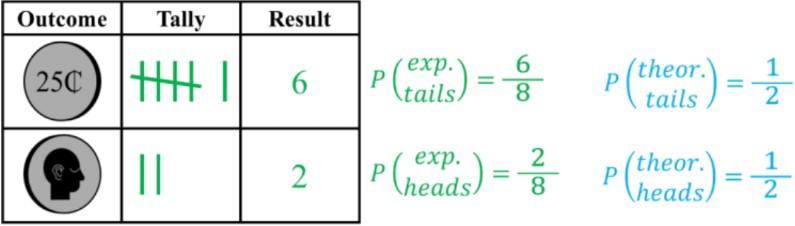
- The experimental (exp.) probabilities do not match with the theoretical (theor.) probabilities.
- The more trials you do, the closer the results should get to the expected probabilities (i.e. doing 10 trials vs. 100 trials vs. 1000 trials, etc.)