Sentiments on statistics in research and academia have rarely been viewed in the positive light – at least at the very beginning. Often failing to disguise itself as anything but “more math”, budding students transitioning into tertiary education have attempted to evade statistics like the plague. A disregard and distaste for statistics however is undoubtedly disturbing for educators and industry professionals all around, particularly among the circles of STEM. How is something so critical in helping students develop quantitative reasoning skills, obtain tools to make inferences, assess limitations, detect errors and uncertainty from data, so that decisions and/or conclusions can be formed, be neglected?
One way statistics has eased into peoples good books is through the happy marriage of computer science and statistics. The world of statistics and computer science have collided and melded together as the practice of statistics has moved onto our electronic devices in the form of programming. Languages like R and Python rank as some of the fastest growing and most used programming languages in the last 5 years. The use of R has grown particularly in academic circles for statistical computing is a well sought out skill and proficiency in R or Python is now desired by many employers especially for those who are pursuing careers in STEM. Statistical tests have come a long way since the beginning and harnessing the power and utility of computers will only see it advance and influence others more rapidly and efficiently.
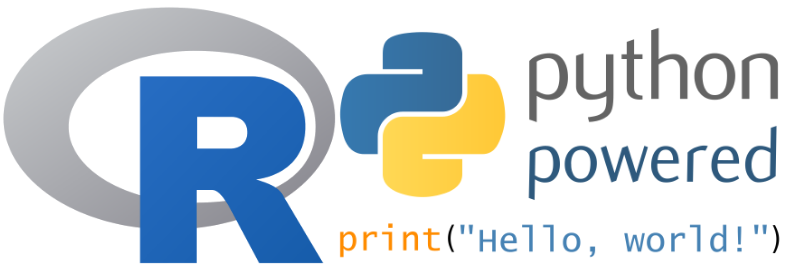
Another way these bad vibes are being countered is the early inclusion of statistics to educational curriculums. In the USA, statistics has been introduced as one of the core components of K-12 Mathematics, highlighting the importance of the learning mathematical skills of induction, deduction, and communication of data. Such practices seem promising as this year alone we should have hit a 50% increase (approximately 200,000 individuals) of professional statisticians entering the workforce. Learning statistics earlier should provide educators a chance to cultivate an earlier appreciation of statistics and corresponding valuable analytical skills. Educators should not provide students with the illusion that pursuing a career in geology or nursing will end all affairs with statistics because the truth is the pervasiveness of data analysis is far-reaching and only increasing in importance as we rely on the data to advance into the future.
So having chosen to embrace statistics, where and who can we expect to be at the frontier of statistics? The truth is many of you will be at the heart of it before knowing it. As emphasized earlier, statistics is an interdisciplinary study. While often highlighted in sciences, it becomes absolutely relevant and paramount whenever there is a need for research and development. We ask questions, seek for improvements, develop new concepts and need a way to answer or see how these ideas come to life. The next step is to then perform experiments, develop prototypes, run tests, all the while tracking results, recording data. Statistics finally comes into play, helping you assess levels of uncertainty, % of success, project growth or sales rates, where to build houses, or mine Gold. Such is the nature of research and development that involves the application of scientific methods, processes, and systems in order to evaluate and interpret data. Data-driven-statistical- research now forms a fundamental piece of the puzzle when innovating, creating or attempting to progress forward – be it in medicine, academia, business, Information Technology, medicine, economics, or construction.
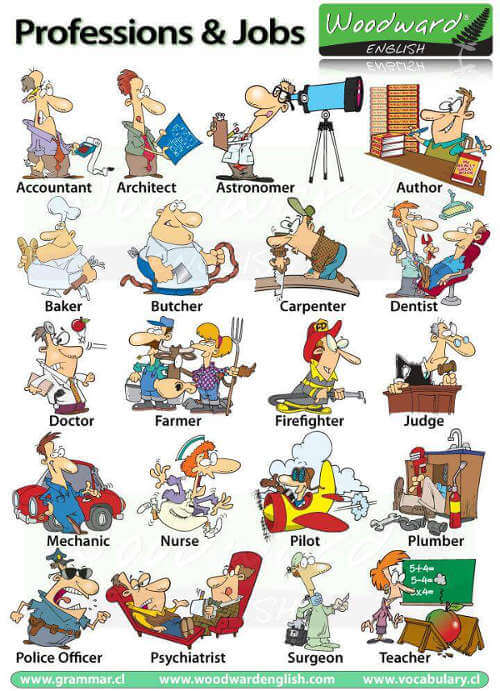
For example, a biostatistician may be involved in researching the rate of HIV spread and invasion throughout sub-saharan Africa to help identify the countries that will be hit the hardest. In medicine, statistical research may take the form of equivalence testing to compare, improve and examine the effectiveness of new drugs to aid depression. Astronomers may utilize statistical models to support research on the expansion of the universe, while an actuary may look for statistical models to predict risk of financial investments or business expansion. Mechanics and automotive industrialists can apply statistics to constantly improve the quality of their product by constantly minimizing the level of errors in the performance of their product. Perhaps a more familiar example is the collation of government statistics. For years, governments have gathered a wealth of enormous datasets and utilized the power of statistics to inform decisions and research improvements on housing, income, unemployment, minimum wage, healthcare, and education services.
So why is it so important to pair scientific research with the use of statistics?
1. Informs methods on data collection
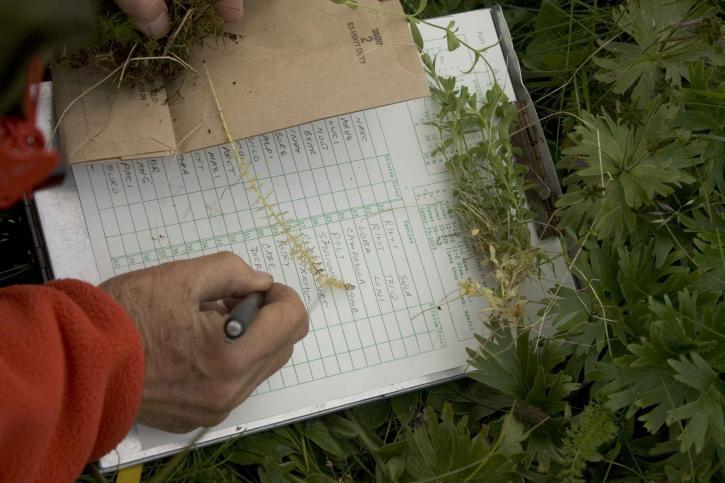
By pre-emptively identifying the statistical test(s) you want to employ to help answer your research question(s), hopefully you know what sort of data needs to be collected. Where statistics comes in handy is helping you identify key aspects you may not have considered in your chosen methods of data collection. Such may come in the form of identifying an additional variable of importance to collect data on. Another pitfall statistics can help you avoid is that of pseudoreplication. Pseudoreplication is particularly dangerous for several reasons: Firstly, it paints a false image of how large a sample size is and ignores the need for “true” replicated treatments (when applicable). Sample sizes are important as they determine the power of your statistical tests and therefore the confidence and scope of your conclusions based on the statistical results. Secondly it fails to highlight that some variables may not be independent. This may mask the true effects of the variables that you wish to be examining independently. Sampling bias can also be avoided when considering the statistical test you hope to use: for example research on the occurrence of domestic violence in households should investigate low-income, middle-income, and high-income neighbourhoods.
“To call in the statistician after the experiment is done may be no more than asking him to perform a post-mortem examination: he may be able to say what the experiment died of.”
— Ronald Fisher
2. Used to support or negate a hypothesis
Without statistical tests there would be no objective way to show whether the data are in support or in disagreement of research questions. Since the burden of evidence (for or against) lies in results of statistic tests, without the use of statistics in research, we would be buried in unknowns, more questions, open-ended conclusions, and more data than we can handle! Without statistical research, we would be unable to credit new discoveries, answer new questions, and confidently advance with new developments. Statistical tests form the basis on each we can trust what the data is saying and make sense of what the raw, volumes of data are communicating.
3. Seeks out uncertainty, errors, and outliers in the data
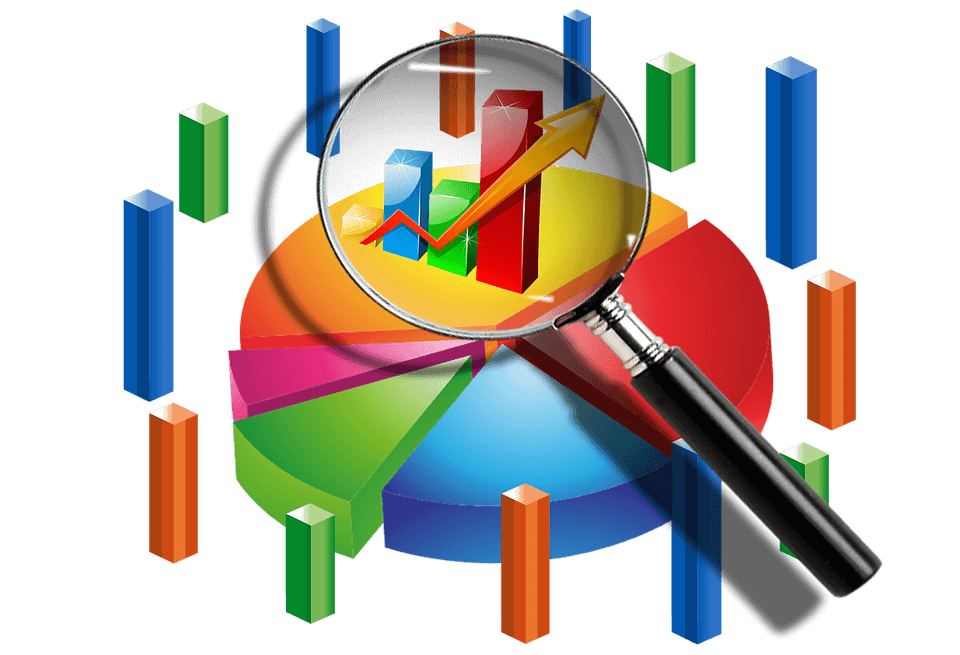
Data is rarely squeaky clean and more often than not, data is messy, ugly and incomplete: Such is the nature of sampling data, there are answers people do not answer completely, truly, or circumstances beyond our control that prevent us to collect all the data points we desire: e.g. an inaccessible village of HIV+ patients trapped in a war zone, the premature death of chicks in a nest, apparatus failure, or the sudden crash in stocks. Truth of the matter is there is no way to collect ALL data points – this is where inferential statistics saves the day. Beyond those limitations, at the very minimum there is human error in data sampling or collection and with every tool, a measure of uncertainty. Errors can also arise due to uncontrollable circumstances as aforementioned, or due to a limitation of a statistical test. These errors can be accounted for to some degree in statistical models and tests so that we can cut through all the noise and assess our hypotheses honestly.
Using statistics can help us map out those outliers, identify the levels of uncertainty in our results, and help us deal fairly with those errors. No statistical test is perfect and neither is any dataset. Statistics allows us to draw conclusions openly by realizing these limitations from the start.
4. Aid interpretation, summarization, and communication of datasets:
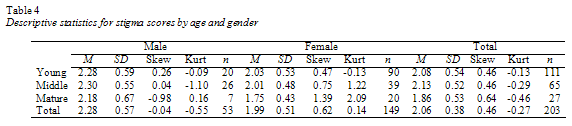
Having utilized the appropriate statistical test, fair and objective conclusions, implications, can now be interpreted from the dataset. Statistical tests provide us with the means to interpret the dataset accurately so that we can make unbiased decisions on how to proceed knowing what the data is saying. It also guides the way we communicate our results and calls for us to defend why these statistical tests were chosen and how we arrived at our explanations based on a series of numbers. Statistics are also a great way of communicating and condensing large datasets into digestible, bitesize pieces of information easily understood by the masses. These summary statistics are helpful in providing people with an immediate idea of the big picture and whether your conclusions are valid.
5. Multivariate statistics and modelling
Without statistics we would be unable to tease apart the multitude of effects that may be influencing our dependent variable. Furthermore we would not be able to identify which factors are working in conjunction to produce a compounded effect on our dependent variable. Statistical modelling helps us deal with our multivariate statistical questions so that we can assess hypotheses from every possible angle. So for example, how do we know that domestic violence in neighbourhoods of various levels of income are not also affected by ethnicity, religion, and level of education? Some of the factors may be intertwined and using statistics helps us tease apart these details.
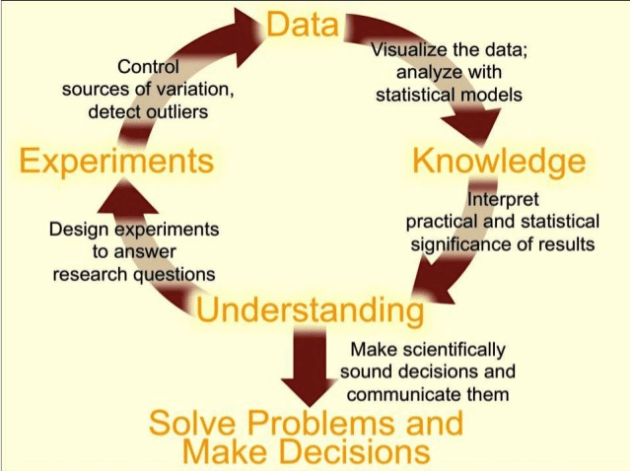
With all that being said, it is worth pointing out that statistics can’t solve everything and anything under the sun perfectly. Statistical tests/models are flawed and in themselves have limitations in the way they were designed and formulated. Even using the wrong statistical test can lead to serious erroneous conclusions and overlook the data completely. Statisticians have thus tried to create helpful guides, books, charts and keys to help advise students and working professionals alike how to identify the appropriate tests/models to apply to their data. These resources should help students be more vigilant and aid the appropriate use and digestion of statistics. Combined with a more positive outlook on statistics, early exposure, an abundance of tools, and the knowledge of a ubiquitous need for statistics in all forms of research and development, there is hope that statistics will be shunned no more. Surely if plants can sense and harness the value of statistics, so can we.
Remember that all models are wrong; the practical question is how wrong do they have to be to not be useful.
— George Box & Norman R. Draper